- Tail Watching
- Posts
- I took random trades for a year. Here's how my portfolio performed
I took random trades for a year. Here's how my portfolio performed
"I know nothing about investing. I'm better off making investment decisions with a coin flip!"
These were the exact words that a friend said to me in 2017.
What if he did use a coin as his investment advisor?
Would he really be better off?
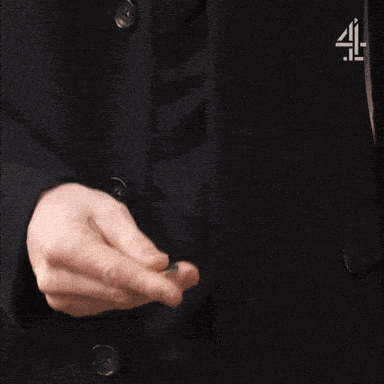
Is price a random walk?
The random walk hypothesis suggests that changes in asset prices follow a "random walk", and are independent of one another. This suggests that past price movements cannot be used to predict future price paths. This is consistent with the efficient-market hypothesis, which suggests that asset prices fully reflect all available information. Trend followers would likely believe that the market could be a random walk with a drift. Some would go as far as to say that the markets are predictable to a certain degree.
A few years back, I embarked on a quest to explore the randomness of price distributions, in an effort to understand the degree to which stochastic price drifts could be estimated.
I started by looking at the distribution of EUR/USD prices across different hours of a trading day:

Fig. 1: EUR/USD price distributions across a trading day (GMT+2), for the last 5,492 trading days
The first few insights that I derived were:
The average range of an hourly candle was 23 pips
The average wick size of an hourly candlestick was 6-7 pips, giving an average hourly retracement of 30%
Volatility picked up pace after 0900 hours, due to the closing of the Sydney and Tokyo trading sessions and the opening of the London and New York sessions
There are more exciting insights you could find in Fig. 1. Do you see them?
To help me with visualizing price movements throughout a trading day, I created an overlay of past price distributions for the last 100 days:

Fig. 2: Overlay visualization of price distributions for the last 100 trading days.
What do you see?
Dart-throwing monkeys & random portfolios
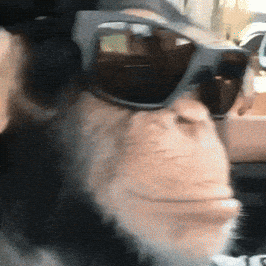
In 1988, the Wall Street Journal created a contest to test the random walk hypothesis by pitting professional investors against "dart-throwing monkeys". The professional investors had the last laugh when their stock picks outperformed the monkeys. I thought it would be interesting to re-create this experiment with the currencies market. With a twist, of course.
I ran a trading algorithm for a year, with random entries, in the name of research.
I named it the SWAN algorithm: Sleep Well At Night (yes, with reference to our all-time favourite black swan event!).
Here were the rules I set for my year-long experiment:
Starting account balance: USD 5,000
Currency pairs: EUR/USD
Trade entry: Random (buy or sell) trades, at random hours of the week
Trade exit: Aha! Here lies the twist that I was telling you about. I get full control of when, how, and which method I use to close my open positions. This includes the use of breakeven points, trailing stops and time-based exits.
Maximum drawdown: 20% of starting balance. This means I would close all open positions if my floating losses exceeded USD 1,000 at any point in time.
To optimize my trade executions, I ran the algorithm with the following configurations:
Trading platform: MetaTrader 4
Trading server: Virtual private server (GMT+2)
Latency: 1-3 milliseconds
Chart: EUR/USD M1 chart
I left the algorithm on autopilot, with zero human intervention (except for restarting the terminals over the weekend).
Sleeping well at night
Full disclosure – there were nights where a bevy of black-feathered swans chased me down in my sleep.
But I digress.
Here's how the SWAN algorithm did in 2018:

Fig. 3: Summary of trades taken by the SWAN algorithm in the year of 2018.
The SWAN algorithm made 4.74% with a 5.45% drawdown, giving it a return over maximum drawdown (RoMAD) of 0.87. 318 trades were opened in 2018, of which 53% were longs and 47% were shorts. Win rate stood at 58% - not bad at all for random entries!

Fig. 4: Equity curve & monthly distribution of returns made by the SWAN algorithm for the year of 2018.
Like in life, the SWAN algorithm had its fair share of ups and downs. It saw red in the months of January and August, but managed to average a daily return of 0.01% and a monthly return of 0.39%.
Was it luck?
The truth is, I would only find out if luck was my only trading edge after a significant number of trades have been made. I know for a fact that luck could play an important part in trading and investing. I did, however, spend a significant amount of time identifying the best possible exit strategies based on what I knew about price behaviour. Even though the entries were random, I had full control of the exits. To be precise, there were 6 exit strategies built into the SWAN algorithm. To limit exposure, trades were closed if none of the exit criteria were met for a pre-determined amount of time.
Would I do this again? Absolutely, but not with random entries.
There is great upside potential by optimizing high probability entry points with refined exits, akin to building a balanced basketball team with stellar attack and strong defence. With a well-diversified portfolio of uncorrelated assets, short term losses could be overcome with long term positive expectancy.
Reply